Deep learning is the key to unlocking value from large sets of unstructured data. Some well-known deep learning applications include speech and image recognition and natural language processing. But, there are countless other applications in medicine, science, business, and more. Deep learning has the potential to help businesses with forecasting and predictions. However, that doesn’t mean deep learning should be used for everything.
Users must consider the costs first. Deep learning is more expensive than its shallow AI alternatives since it has to work with massive amounts of unstructured data. Hardware requirements may include high-end GPUs, multiple servers, and a hybrid infrastructure that allows teams to extend their resources as needed.
Despite the costs, the rewards of employing deep learning can far outweigh the price. To determine your need for deep learning, consider the following questions:
- Do you need a high level of accuracy?
- Will you have access to or have the budget for GPU resources?
- Does your data set contain thousands or millions of data points?
If you answered yes to these questions, you might be a good candidate for deep learning. Keep in mind that this is not an exhaustive list, merely a starting point. Once you’ve decided to move ahead with deep learning, you’ll need to ensure your hardware is capable of running the algorithms efficiently.
Hardware Requirements for Deep Learning Jobs
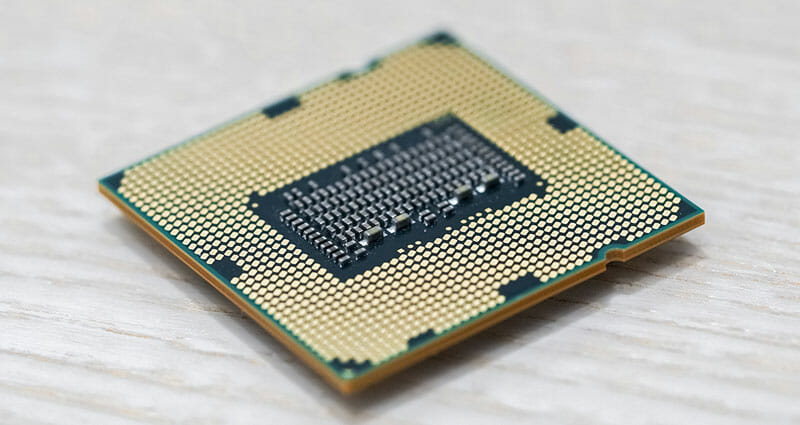
To get the most out of every dollar, it’s important to choose your deep learning hardware with precision. Failing to match hardware with the proper type of deep learning can result in excessive spending and inefficiencies. Consider three hardware components that directly impact the effectiveness of your infrastructure.
1. GPU Hardware Is the Standard in Deep Learning
Even with the rise of accelerated hardware like TPUs, GPUs are still the hardware of choice for deep learning, in no small part due to their flexibility. Jobs that require a lot of neural computation need GPU hardware as a CPU won’t provide the parallel processing capabilities required to run algorithms in a timely manner.
It’s important to note that teams must prioritize the quantity and quality of their GPUs. Underpowered hardware will provide diminishing returns at scale, so it’s not practical for teams to add these types of nodes to their network indefinitely. In the long-term, it’s more efficient from a power consumption and performance perspective to invest in top-of-the-line equipment.
2. CPU
The prevalence of GPU usage in deep learning causes some to believe that they should always be used. However, some jobs do better with the multi-threading capabilities of a CPU, especially for training simulations in physics and molecular docking applications. Even when GPUs are primarily used, solid CPU hardware can speed up system orchestration — although it’s not as critical to invest in high-end CPU components.
While some jobs do lend themselves to CPU capabilities, it may be possible to rewrite algorithms to use GPU hardware. Using a GPU in this way can significantly improve speed over its CPU counterpart.
3. Memory and Storage
After fine-tuning your processors to match your deep learning needs, your biggest bottleneck is storage. Deep learning depends on large amounts of data, possibly millions of data points. Low latency storage ensures that deep learning isn’t held back due to sluggish storage drives. Modern SSDs provide a great balance between speed and storage density.
Another consideration is RAM, especially when dealing with large data sets. Typically, you’ll want to have more RAM than GPU memory — how much more will depend on the deep learning job you’re trying to complete. Thankfully, RAM is unlikely to break the bank. In fact, quantity is more important than procuring the highest clock speeds.
Start Optimizing Your Deep Learning Infrastructure
Fine-tuned deep learning hardware will speed up the time it takes for you to get results from your data. Hence, you’ll achieve faster breakthroughs and speed up your ROI. However, supply chain expertise, deployment challenges, and a lack of time may be holding you back. We can help.
Equus provides full-lifecycle hardware support for deep learning and high-performance computing. We’ll help you design, deploy, and maintain your hardware so that you can focus on answering the questions you’re using deep learning for. Talk to an expert to discuss your deep learning needs today.